The CSSI-GW Project aims to improve the understanding and representation of atmospheric gravity waves using
high-resolution observations, simulations, and machine learning. The project also aims to build and support a community-driven
cyberinfrastructure for data and codes focused on gravity waves research.
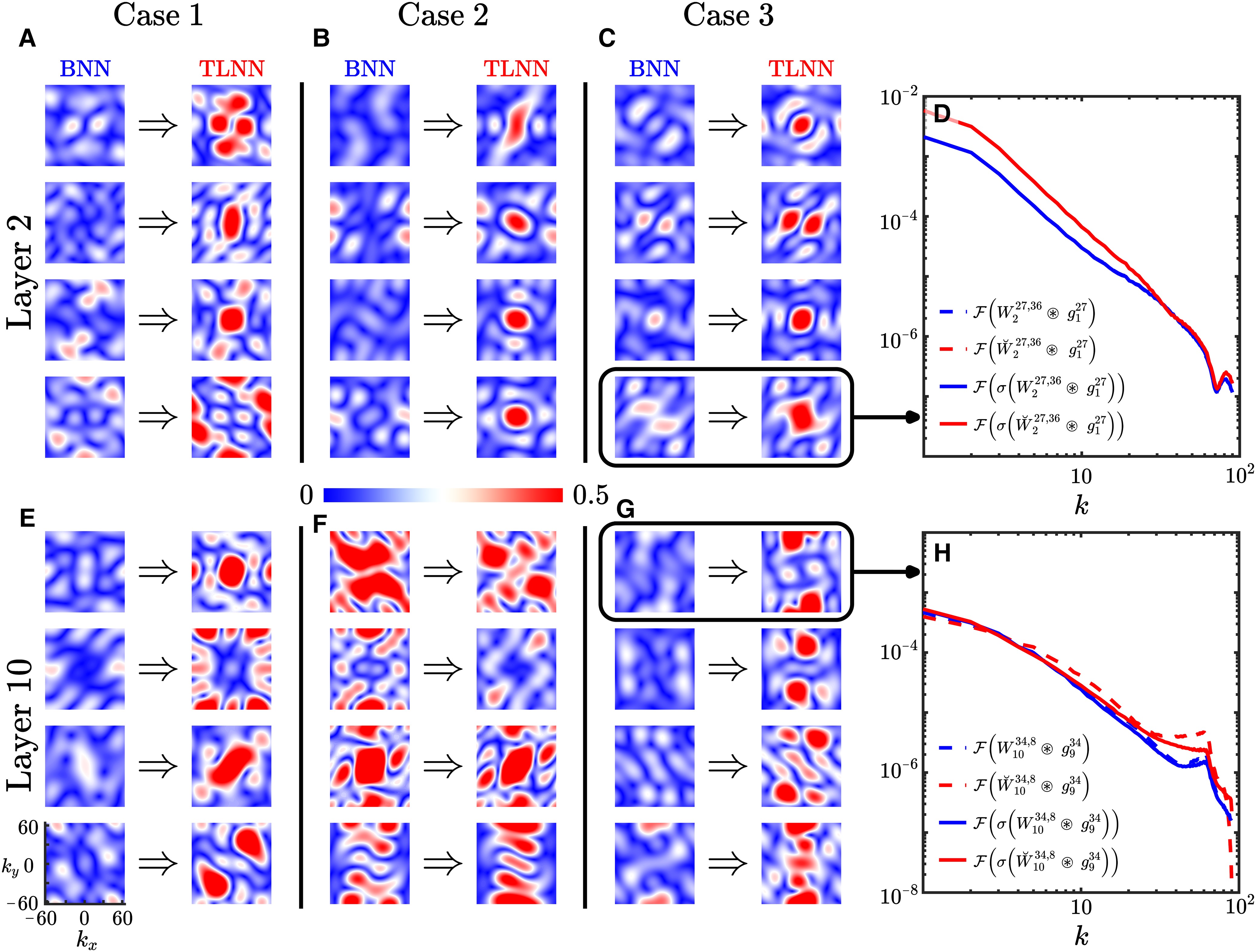
Recent publications
Key Points:- We present novel analyses and a framework addressing (1) how to re-train NNs? and (2) what physics are learned during TL? for a broad range of multi-scale, nonlinear, dynamical systems.
- Our approach combines spectral (e.g. Fourier) analyses of such systems with spectral analyses of convolutional NNs, revealing physical connections between the systems and what the NN learns (a combination of low-, high-, band-pass filters and Gabor filters).
- Integrating these analyses, we introduce a general framework that identifies the best re-training procedure for a given problem based on physics and NN theory. As test case, we explain the physics of TL in subgrid-scale modeling of several setups of 2D turbulence. Furthermore, these analyses show that in these cases, the shallowest convolution layers are the best to re-train, which is consistent with our physics-guided framework but is against the common wisdom guiding TL in the ML literature.
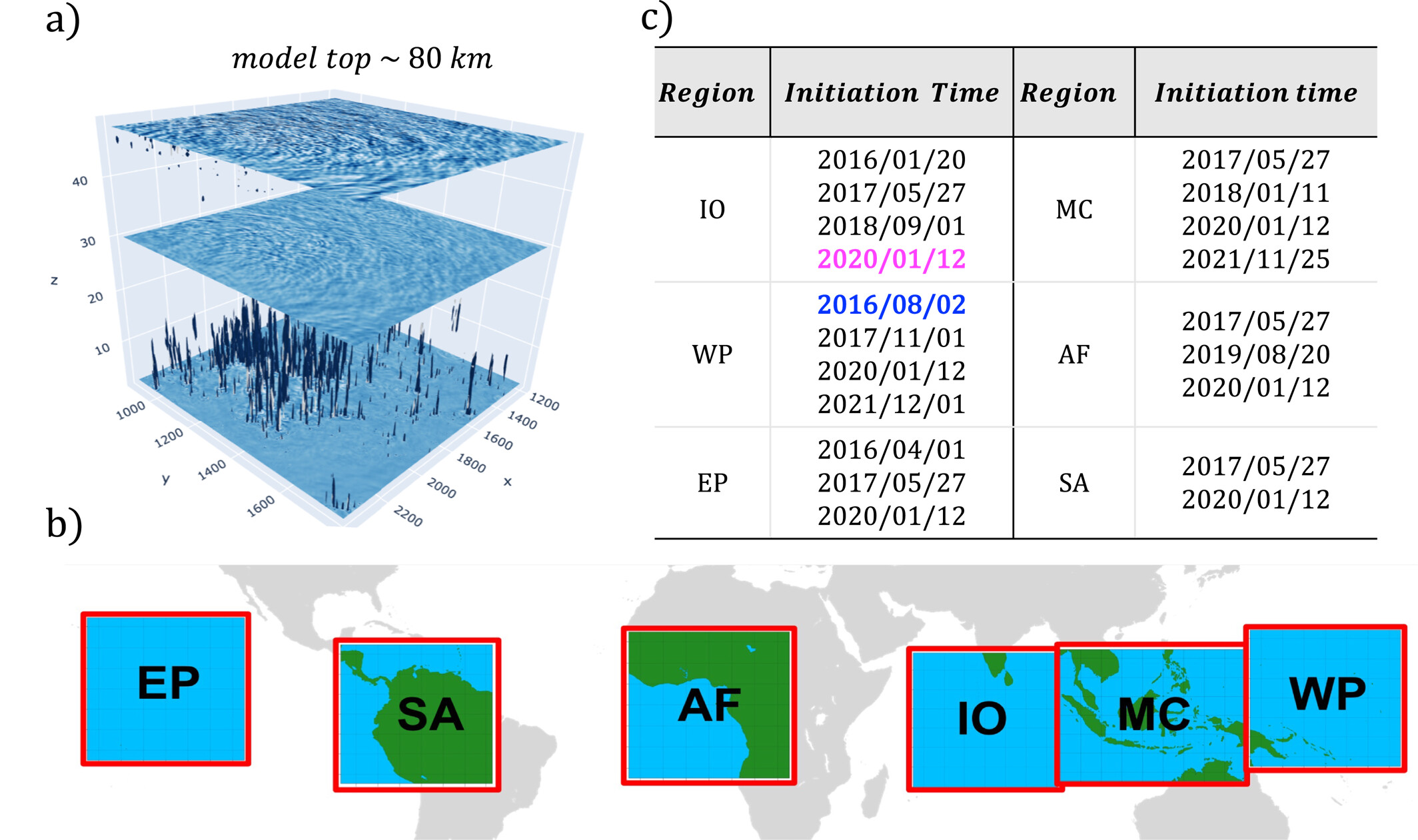
Recent publications
Key Points:- In a library of weather research and forecasting model simulations, we compare methods for estimating 3D gravity wave drag force that are un- and under-resolved by general circulation models.
- For drag associated with vertical fluxes, different methods agree on time- and zonal-mean but not on instantaneous spatiotemporal patterns.
- Drag associated with horizontal fluxes is significant but is very sensitive to the estimation methodology.
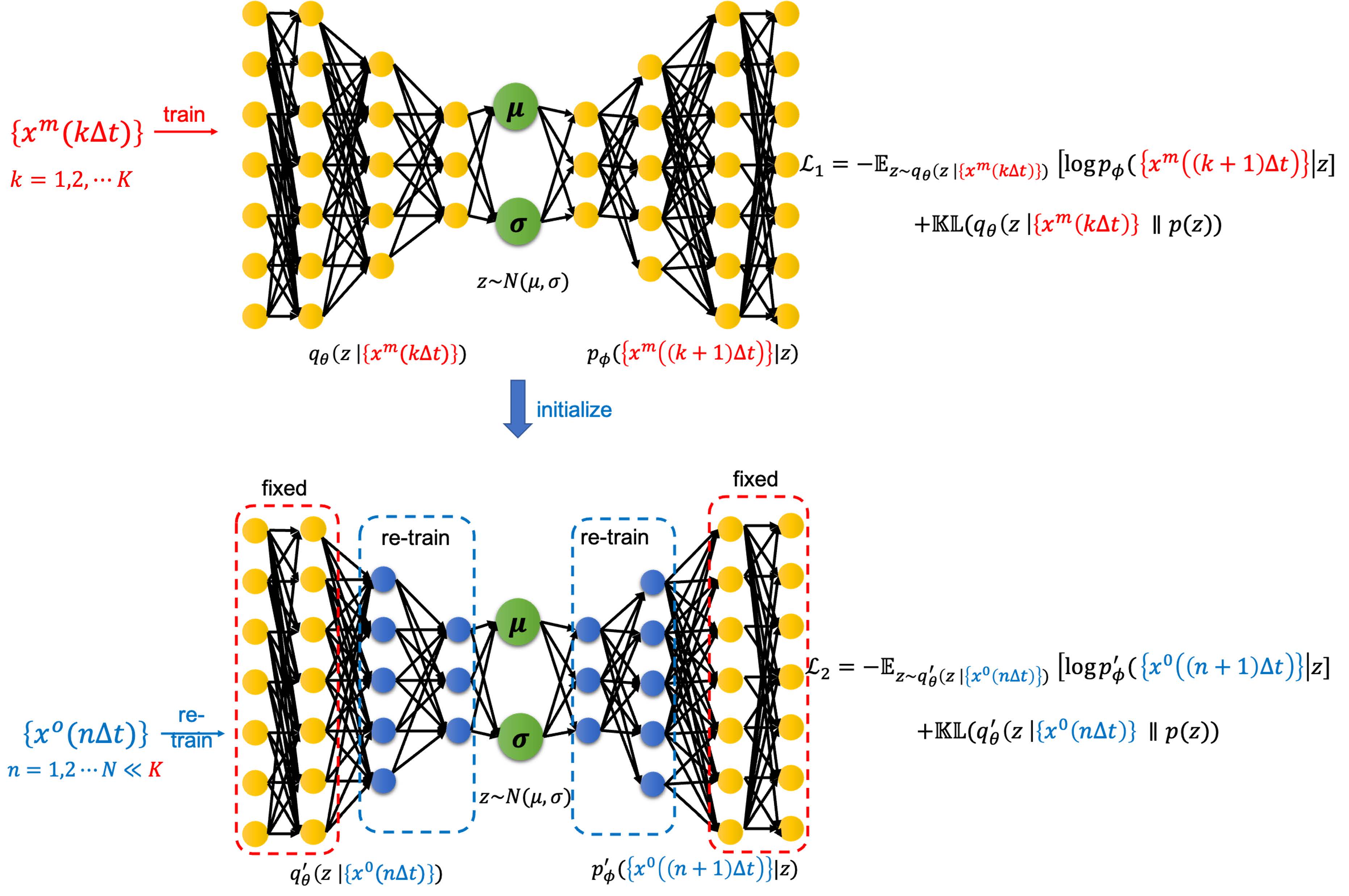
Recent publications
Key Points:- We propose a convolutional variational autoencoder-based stochastic data-driven model that is pre-trained on an imperfect climate model simulation from a 2-layer quasi-geostrophic flow and retrained, using transfer learning, on a small number of noisy observations from a perfect simulation.
- This re-trained model performs stochastic forecasting with a noisy initial condition sampled from the perfect simulation.
- Our ensemble-based stochastic data-driven model outperforms a baseline deterministic encoder-decoder-based convolutional model in terms of short-term skills while remaining stable for long-term climate simulations yielding accurate climatology.
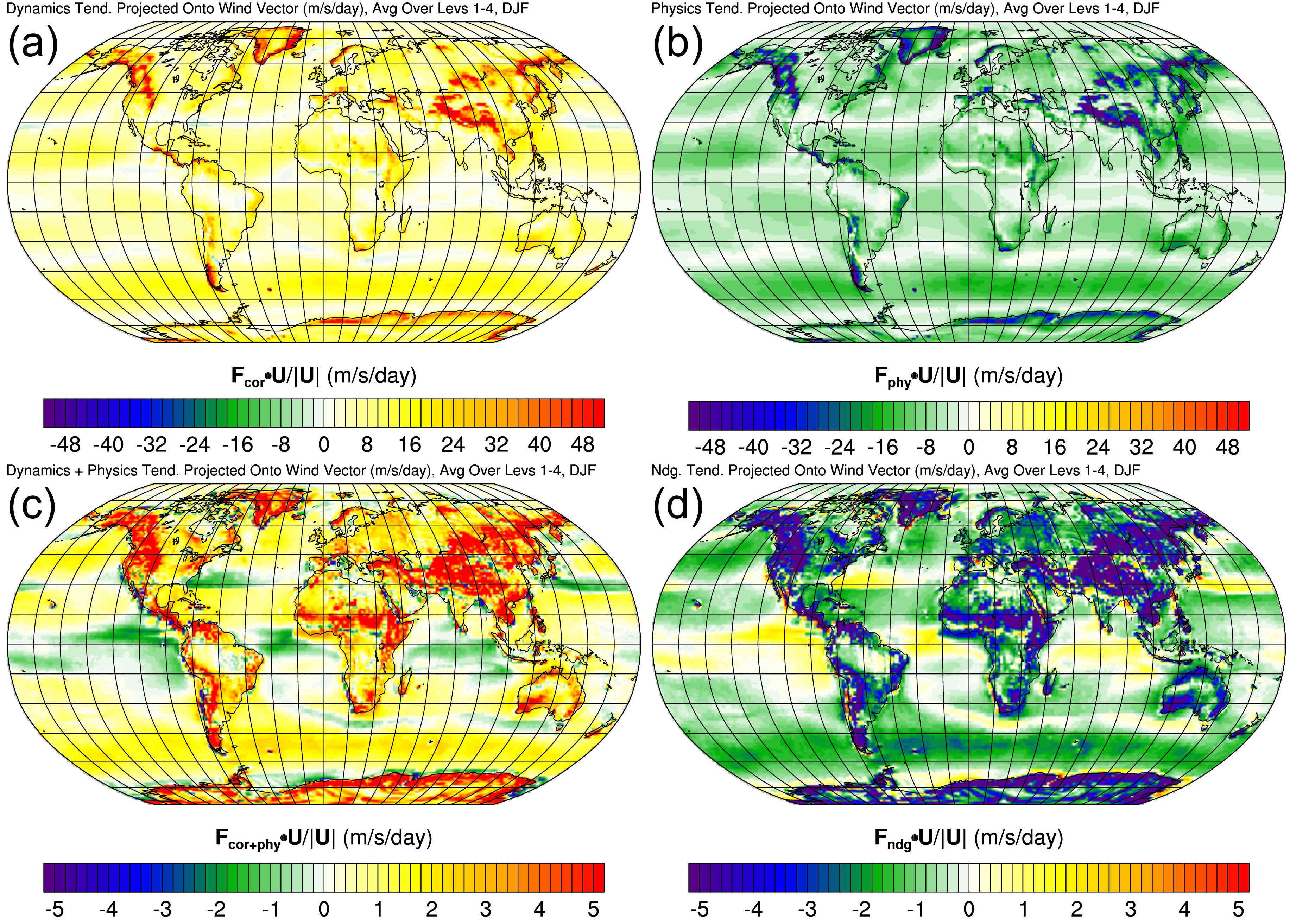
Recent publications
Key Points:- Nudging tendencies, or nudging forces per unit mass, depend strongly on the nudging time scale chosen (i.e. are ∝ τ−1).
- Nudging tendencies cannot be quantitatively interpreted as errors in total (dynamics + physics) model forces on the atmospheric fluid.
- Still, nudging tendencies can be useful for identifying model errors, even if only qualitatively.
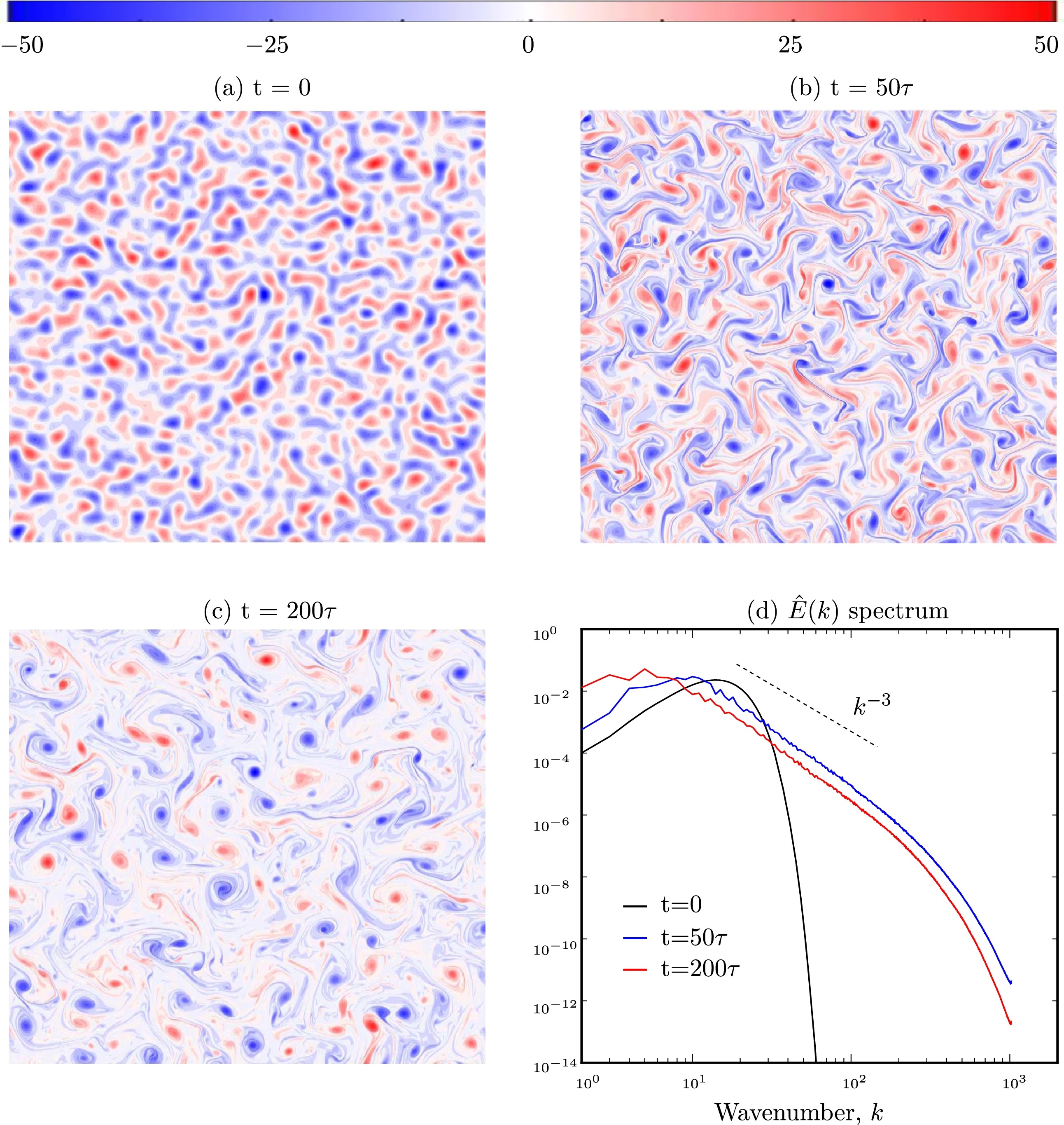
Recent publications
Key Points:- A deep learning-based, non-local data-driven SGS model is introduced.
- This SGS model accurately captures the effect of small-scale structures.
- Transfer learning enables the SGS model to extrapolate to more turbulent flows.
- Resulting LES model is stable and generalizable with accuracy superior to baseline LES.
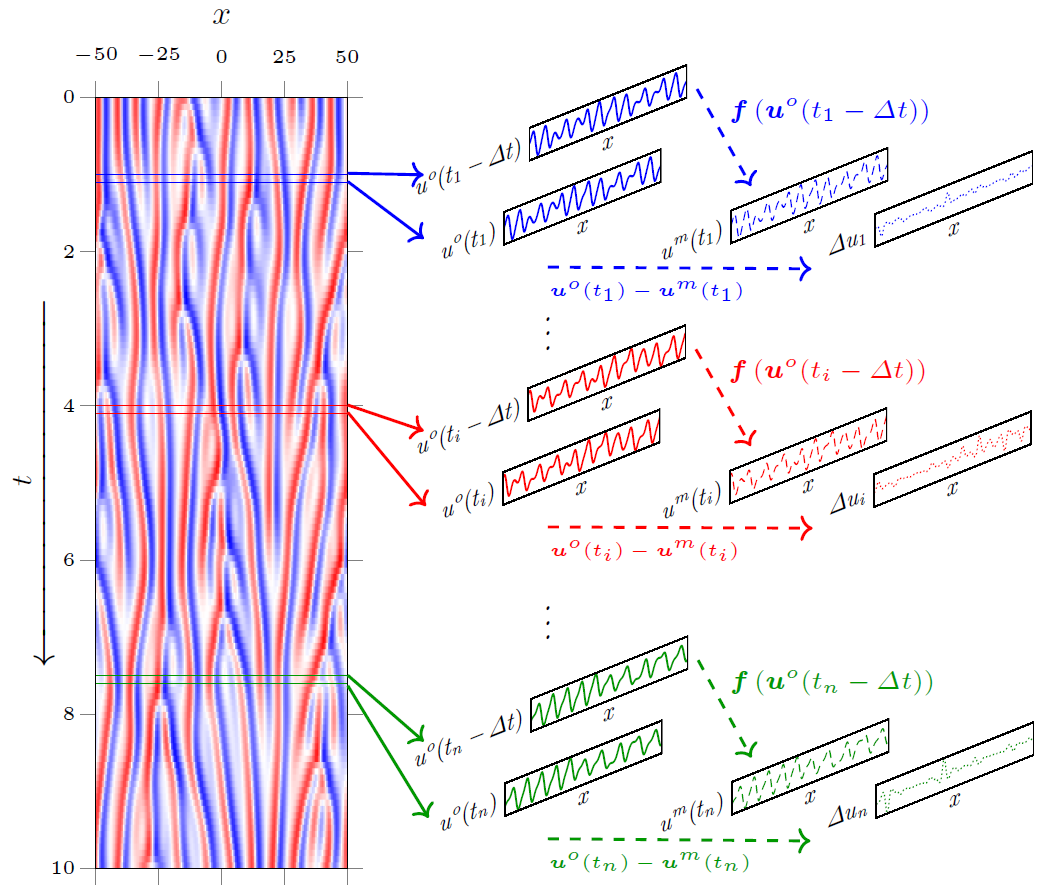
Recent publications
Key Points:- • We introduce a framework named MEDIDA: Model Error Discovery with Interpretability and Data Assimilation.
- • MEDIDA only requires a working numerical solver of the model and a small number of noise-free or noisy sporadic observations of the system.
- • The framework proposed here integrates machine learning (ML) and data assimilation (DA) techniques to discover close-form, interpretable representations of these model errors.
- • This framework can leverage the ever-growing abundance of observational data to reduce the errors in models of nonlinear dynamical systems, such as the climate system.
- • Using the chaotic Kuramoto–Sivashinsky system as the test case, we demonstrate the excellent performance of MEDIDA in discovering different types of structural/parametric model errors.
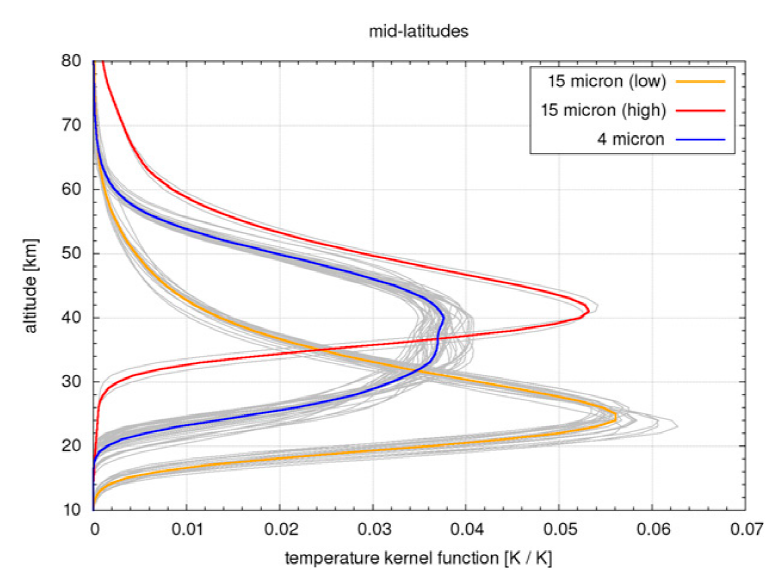
Recent publications
Key Points:- • Four state-of-the-science numerical weather prediction (NWP) models were used to perform mountain wave (MW)-resolving hindcasts over the Drake Passage of a 10-day period in 2010 with numerous observed MW cases. All models reproduced observed middle-atmosphere MWs with remarkable skill.
- • This study had three purposes: to quantitatively evaluate how well four state-of-the-science weather models could reproduce observed mountain waves (MWs) in the middle atmosphere, to compare the simulated MWs within the models, and to quantitatively evaluate two MW parameterizations in a widely used climate model.
- • Meridionally propagating MWs can significantly influence zonal winds over the Drake Passage. Parameterizations that handle horizontal propagation may need to consider horizontal fluxes of horizontal momentum in order to get the direction of their forcing correct.
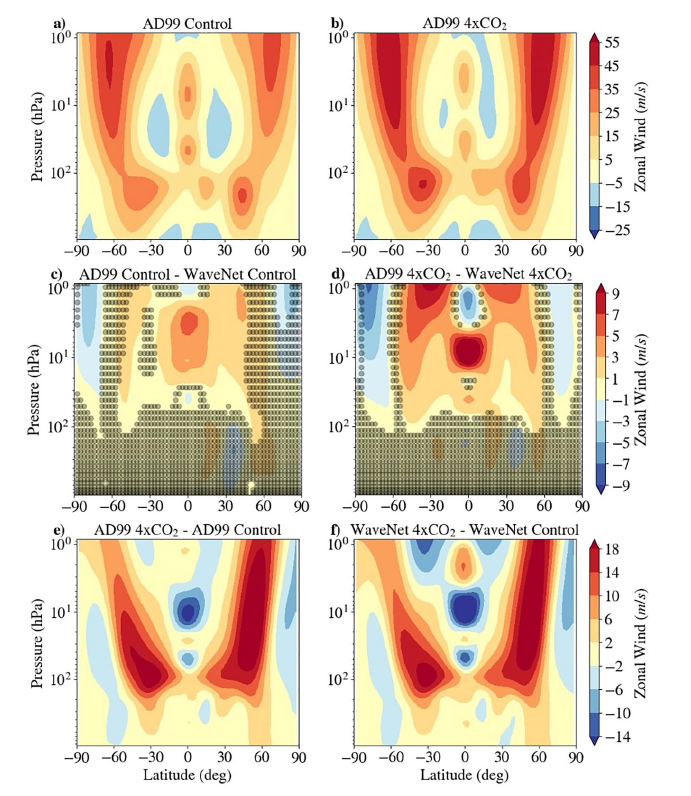
Recent publications
Key Points:- • We present single-column gravity wave parameterizations (GWPs) that use machine learning to emulate non-orographic gravity wave (GW) drag and demonstrate their ability to generalize out-of-sample.
- • A set of artificial neural networks (ANNs) are trained to emulate the momentum forcing from a conventional GWP in an idealized climate model, given only one view of the annual cycle and one phase of the Quasi-Biennial Oscillation (QBO).
- • We investigate the sensitivity of offline and online performance to the choice of input variables and complexity of the ANN.
- • When coupled with the model, moderately complex ANNs accurately generate full cycles of the QBO.
- • When the model is forced with enhanced CO2, its climate response with the ANN matches that generated with the physics-based GWP.
- • GThat ANNs can accurately emulate an existing scheme and generalize to new regimes given limited data suggests the potential for developing GWPs from observational estimates of GW momentum transport.